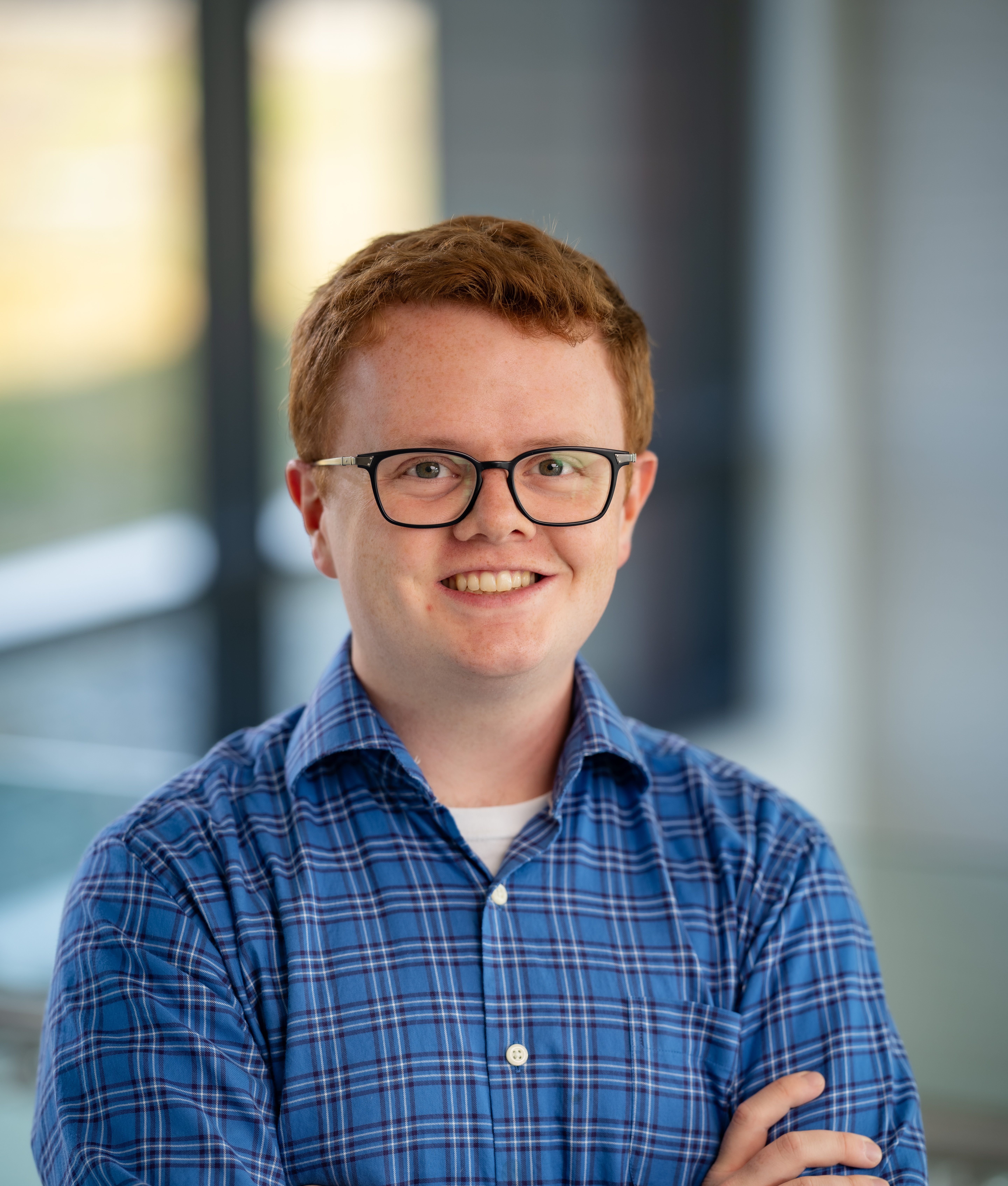
Predoctoral Researcher
Allen Institute for AI
Jacob Morrison
Hi! I'm a predoctoral researcher on the AllenNLP team at Ai2, and I'm advised by Pradeep Dasigi and Jesse Dodge. I received my masters in computational linguistics and bachelors in computer science from the University of Washington, where I was advised by Noah Smith. I've previously worked on code & program synthesis at Google [x], language + vision models at Ai2, and platform health at Twitter, and I also spent a few years as a software engineer at Tableau and Google. See my CV for more details.I'm applying to PhD programs! Feel free to reach out if you're interested in chatting. I'm interested in building broadly capable LMs, and I'll be supported by an NSF Computer Science Graduate Fellowship.
Research
My research is generally focused on making modern language models broadly useful and reliable. I've recently been focused on improving model capabilities through post-training by creating new datasets and evaluations, and improving training algorithms and model architectures. I'm also a strong supporter of open science, and I've contributed to openly released artefacts including Tulu 3, RewardBench, Dolma, and OLMo, OLMo 2, and OLMoE, with more coming soon.
I also spend a portion of my time helping policymakers understand and address the societal impacts of advances in AI, and I regularly engage with policymakers at the local, state, and federal levels. I previously served on the City of Seattle's Generative AI Policy Advisory Group, and I'm currently serving on the Education and Workforce Development Subcommittee of the Washington State AI Task Force.
Awards & Fellowships
- Aug. 2024: ACL Theme Paper Award
- Aug. 2024: ACL Best Resource Paper Award
- Aug. 2023: NSF Computer Science Graduate Fellowship
Publications
2025
2024
- TΓΌlu 3: Pushing Frontiers in Open Language Model Post-Training
-
Holistically Evaluating the Environmental Impact of Creating Language Models
ICLR 2025 π¦ Spotlight Paper π¦
-
OLMoE: Open Mixture-of-Experts Language Models
NeurIPS 2024 Workshop on Efficient Natural Language and Speech Processing (spotlight) π¦ Spotlight Paper π¦ICLR 2025 paper π£οΈ Oral Presentation π£οΈ
-
Merge to Learn: Efficiently Adding Skills to Language Models with Model Merging
Findings of EMNLP 2024 paper
-
SciRIFF: A Resource to Enhance Language Model Instruction-Following over Scientific Literature
arXiv paper
- RewardBench: A Benchmark for Evaluating Reward Models
-
Intentionally Unintentional Speech: Why Generative AI Models Are Not Protected by the First Amendment
First Amendment Law Review (University of North Carolina), Spring 2025 paper
-
Unsettled Law in the Age of Generative AI: Time to Generate New Approaches?
Journal of Law and Technology at Texaspaper
-
A Legal Risk Taxonomy for Generative Artificial Intelligence
arXiv preprint paper
-
OLMo: Accelerating the Science of Language Models
ACL 2024 paper π₯ Theme Paper Award π₯
-
Dolma: an Open Corpus of Three Trillion Tokens for Language Model Pretraining Research
ACL 2024 paper π₯ Best Resource Paper Award π₯